Diffusion-Based Signed Distance Fields for 3D Shape Generation
CVPR 2023
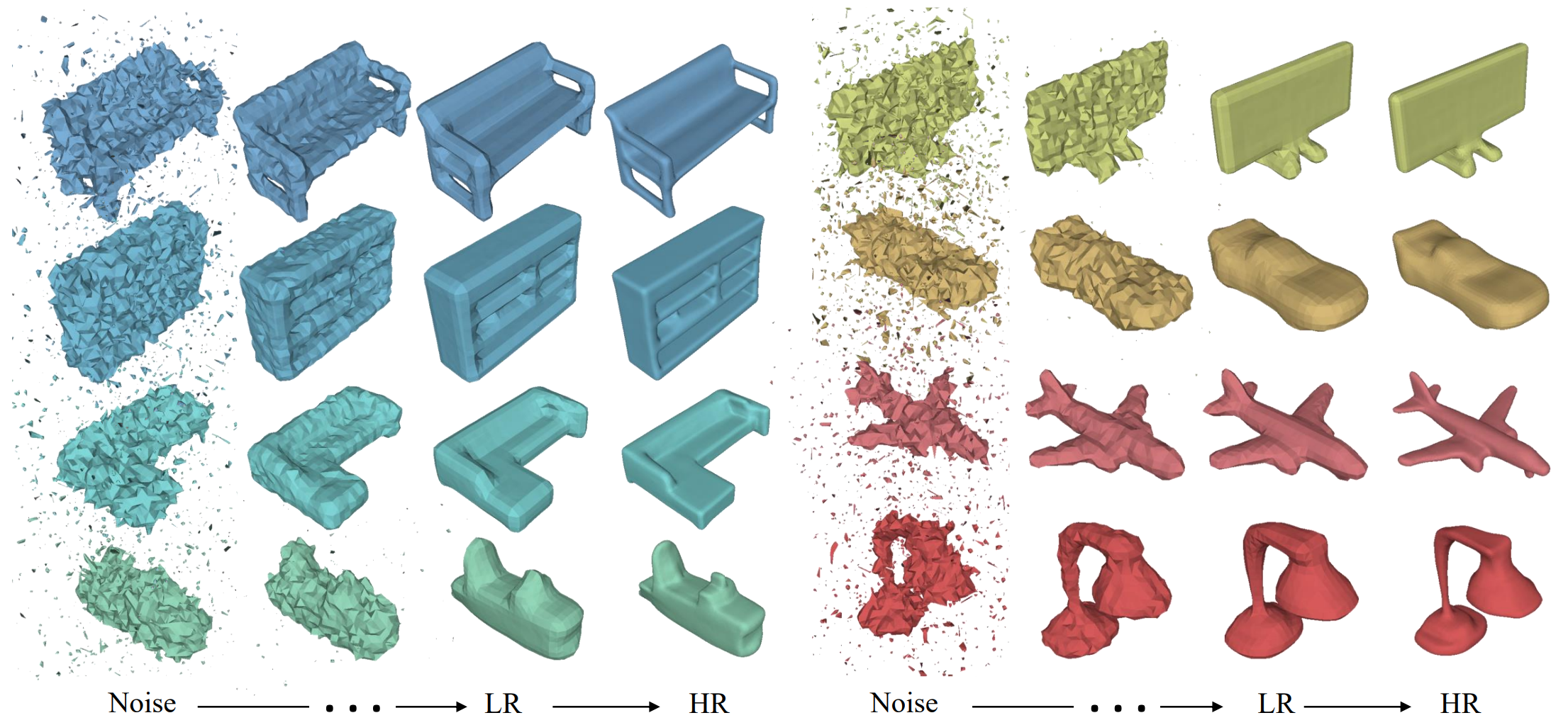
Abstract
We propose a 3D shape generation framework (SDF-Diffusion in short) that uses denoising diffusion models with continuous 3D representation via signed distance fields (SDF). Unlike most existing methods that depend on discontinuous forms, such as point clouds, SDF-Diffusion generates high-resolution 3D shapes while alleviating memory issues by separating the generative process into two-stage: generation and super-resolution. In the first stage, a diffusion-based generative model generates a low-resolution SDF of 3D shapes. Using the estimated low-resolution SDF as a condition, patch-based diffusion model performs super-resolution in the second stage. Our framework can generate a high-fidelity 3D shape despite the extreme spatial complexity. On the ShapeNet dataset, our moedel shows competitive performance to the state-of-the-art methods and shows applicability on the shape completion task without modification.
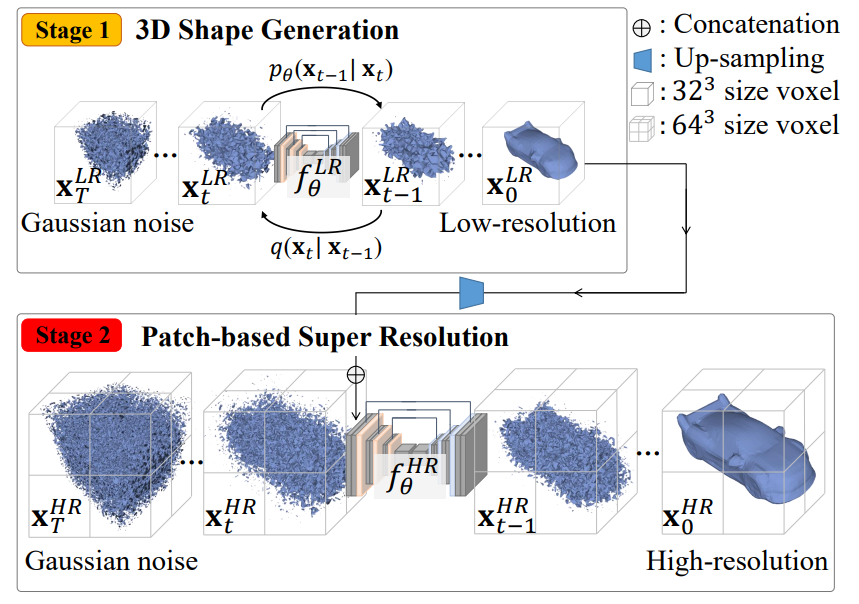
Methodology
An overview of our method. Our method can generate high-resolution 3D shapes in the form of SDF voxels based on the denoising diffusion model (DDM). Our method has two stages: low-resolution coarse shape generation (top of the figure) and shape super-resolution for high-resolution fine shape on the voxel-shaped SDF (bottom of the figure). In the first stage, a diffusion-based 3d generative model learns to create realistic low-resolution 3D shapes. In the second stage, a diffusion-based super-resolution model is trained to upsample the 3D shapes. To alleviate cubic memory issue to handle high-resolution voxel, the second stage model is trained to super-resolve 3D shapes from corresponding high- and low-resolution patches.
Generation Results
Generated 3D shapes from our method trained on ShapeNet dataset. 32, 64, 128 means resolution of voxel-shaped SDF of generated samples.
Shape Completion Results
Our method is capable of generating 3D shapes with conditioned on partial 3D shapes. Using the same condition input, our method can produce various harmonious candidates.
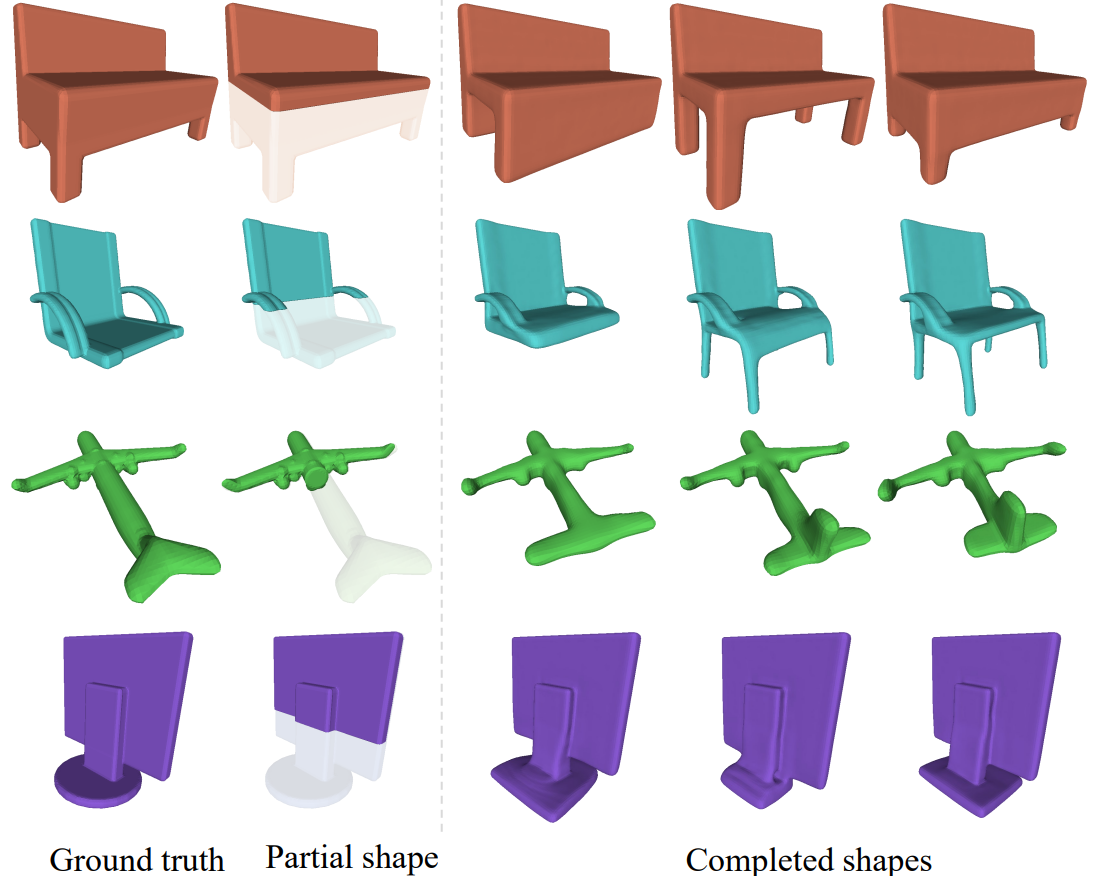
Acknowledgements
This work was supported by Institute of Information & communications Technology Planning & Evaluation (IITP) grant funded by the Korea government (MSIT) (No. 2022-0-00612, Geometric and Physical Commonsense Reasoning based Behavior Intelligence for Embodied AI, No.2022-0-00907, Development of AI Bots Collaboration Platform and Self-organizing AI and No.2020-0-01336, Artificial Intelligence Graduate School Program (UNIST)) and Artificial intelligence industrial convergence cluster development project funded by the Ministry of Science and ICT (MSIT, Korea) & Gwangju Metropolitan City.